In today’s dynamic business landscape, where agility and efficiency are paramount, organizations are turning to machine learning in the supply chain to revolutionize their operations. This transformative technology holds the key to optimizing processes, enhancing decision-making, and ultimately achieving optimal performance in the complex world of supply chain management.
Understanding Machine Learning in the Supply Chain
Machine learning is a subset of artificial intelligence that empowers systems to learn and improve from experience without explicit programming. Within the context of the supply chain, machine learning algorithms can analyze vast amounts of data, identify patterns, and make predictions or decisions with minimal human intervention. This capability can be harnessed across various supply chain functions to drive efficiency and competitiveness.
Demand Forecasting and Inventory Management
One of the most critical challenges in supply chain management is predicting demand accurately. Machine learning algorithms excel in analyzing historical data, market trends, and external factors to provide more accurate demand forecasts. By leveraging machine learning for demand forecasting, businesses can optimize their inventory levels, reducing the risk of overstocking or stockouts. This not only improves customer satisfaction by ensuring products are readily available but also minimizes carrying costs and maximizes profitability.
Route Optimization and Logistics
Customer satisfaction hinges on the ability of businesses to meet demands accurately and promptly. This is where demand planning in supply chain management becomes instrumental. Transportation ManagemEfficient logistics and transportation are essential components of a well-functioning supply chain. Machine learning algorithms can analyze real-time data such as traffic conditions, weather forecasts, and transportation costs to optimize routes and delivery schedules. This not only reduces transportation expenses but also enhances delivery speed and reliability. By leveraging machine learning in logistics, companies can achieve cost savings and improve customer satisfaction through timely and reliable deliveries.ent Systems, when seamlessly integrated into the supply chain, assist in predicting demand patterns based on historical data, market trends, and other relevant factors.
Using advanced algorithms and analytics, TMS aids in forecasting product demand with a high degree of accuracy. This ensures that warehouses are well-prepared to fulfill customer demands, minimizing stockouts, optimizing inventory levels, and ultimately improving customer satisfaction.
Warehouse Automation and Robotics
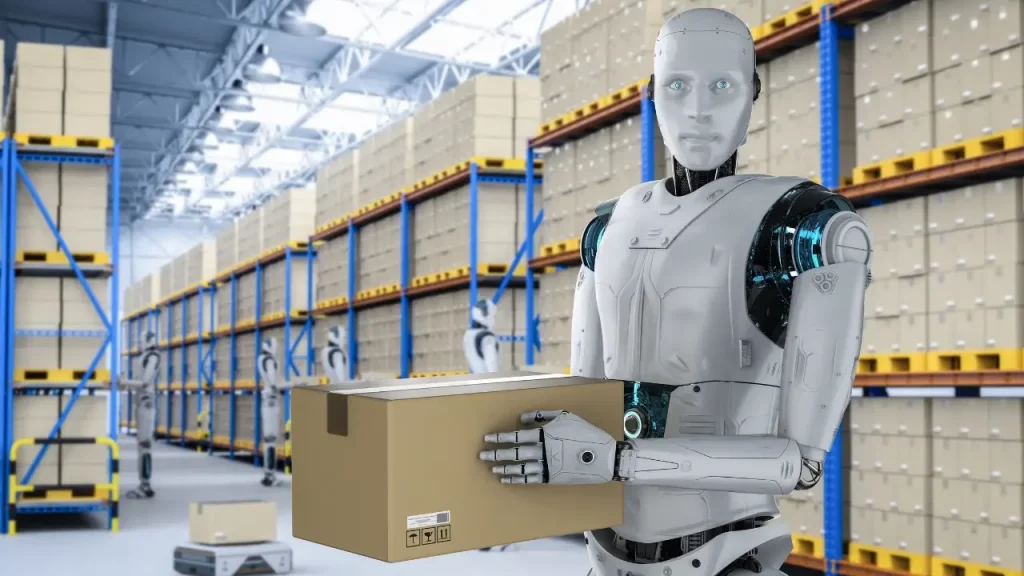
Warehousing is another area where machine learning can significantly impact supply chain efficiency. Machine learning algorithms can optimize warehouse operations by automating tasks such as inventory management, picking, and packing. In conjunction with robotics, machine learning can enhance the speed and accuracy of these processes, leading to faster order fulfillment and reduced operational costs. Automated warehouses powered by machine learning technologies can adapt to changing demand patterns, making them more agile and responsive.
Supplier Relationship Management
Maintaining strong relationships with suppliers is crucial for a smooth and efficient supply chain. Machine learning can be employed to assess and predict supplier performance, identify potential risks, and optimize procurement processes. By analyzing historical data and monitoring real-time factors, machine learning algorithms can help businesses make informed decisions about supplier selection, pricing negotiations, and risk mitigation. This proactive approach to supplier relationship management enhances overall supply chain resilience.
Quality Control and Predictive Maintenance
Ensuring product quality is paramount in supply chain management. Machine learning can contribute to quality control by analyzing data from various sources, including production processes and customer feedback. By detecting patterns and anomalies, machine learning algorithms can identify potential issues before they escalate, enabling timely intervention and reducing the likelihood of defective products reaching the market. Additionally, machine learning can be applied to predictive maintenance, helping businesses optimize the maintenance schedules of machinery and equipment, minimizing downtime and repair costs.
Real-time Visibility and Analytics
Machine learning provides the capability to process and analyze large datasets in real-time, offering unparalleled visibility into the supply chain. By leveraging real-time analytics, businesses can monitor and respond to changing conditions promptly. This visibility enables proactive decision-making, allowing organizations to address issues such as bottlenecks, delays, or disruptions before they escalate. Real-time data analysis is crucial for maintaining agility in the supply chain, especially in today’s dynamic and unpredictable business environment.
Overcoming Implementation Challenges
While the benefits of leveraging machine learning in the supply chain are substantial, there are challenges associated with implementation. Businesses need to invest in data infrastructure, ensure data quality, and address issues related to data privacy and security. Additionally, there is a learning curve associated with understanding and deploying machine learning solutions. To overcome these challenges, organizations should invest in training and collaboration between data scientists and supply chain professionals. Building a cross-functional team that combines domain expertise with data science skills is essential for successful implementation.
Case Studies: Success Stories in Machine Learning Integration
To illustrate the real-world impact of leveraging machine learning in the supply chain, we can look at several successful case studies. Companies like Amazon, FedEx, and Walmart have demonstrated how machine learning technologies can transform their supply chain operations, resulting in increased efficiency, cost savings, and improved customer satisfaction. These case studies serve as inspiration for other businesses looking to embark on their machine learning journey in the supply chain.
The Future of Machine Learning in the Supply Chain
As technology continues to advance, the future of machine learning in the supply chain holds even more promise. Innovations such as the Internet of Things (IoT), blockchain, and advanced analytics will further enhance the capabilities of machine learning algorithms. The integration of these technologies will create a more interconnected and intelligent supply chain ecosystem, enabling organizations to adapt quickly to changing market conditions and customer preferences.
Conclusion
In conclusion, leveraging machine learning in the supply chain is no longer a choice but a necessity for businesses aiming to achieve optimal performance. From demand forecasting to logistics optimization, machine learning can streamline processes, reduce costs, and enhance overall efficiency.
As organizations embrace this transformative technology, they must invest in the necessary infrastructure, talent, and collaboration to unlock the full potential of machine learning in their supply chain operations. By doing so, businesses can position themselves for success in an increasingly competitive and dynamic global marketplace.